Maximizing Returns on AI Model Optimization
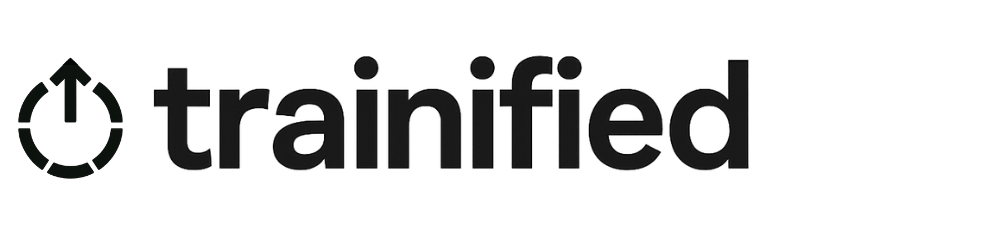
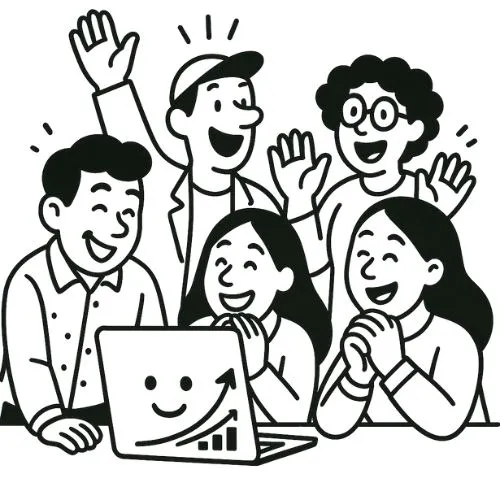
Fine-Tuning ROI Calculator
Inputs
Estimated Tokens: 0
How much you save each time you use the fine-tuned model vs. the base model.
How many times you expect to use the fine-tuned model monthly.
ROI Estimation
Enter valid inputs to see the ROI chart.
Disclaimer: This calculator provides estimations based on generalized assumptions (e.g., ~700 tokens/MB, average query size) and example pricing ($0.008/1k tokens training). Actual costs depend on exact file content, specific model usage, and current OpenAI pricing. Savings per query must be estimated based on your specific use case (e.g., comparing base model cost vs. fine-tuned model cost for typical queries, or value gained from improved performance). This tool is for illustrative purposes only.
Fine-Tune ROI: Maximizing Returns on AI Model Optimization
In the rapidly evolving landscape of artificial intelligence (AI), organizations are increasingly investing in AI models to drive innovation, enhance customer experiences, and streamline operations. However, the development and deployment of AI models can be resource-intensive, requiring significant computational power, data, and expertise. To ensure these investments yield meaningful returns, businesses are turning to fine-tuning, a process that optimizes pre-trained AI models for specific tasks. Fine-tuning not only enhances model performance but also maximizes return on investment (ROI) by reducing costs, improving efficiency, and delivering tailored solutions. This article explores the concept of fine-tuning, its impact on driving ROI, and strategies to optimize the process for maximum financial and operational benefits.
Introduction to AI Model Optimization
AI model optimization is a crucial step in the development of artificial intelligence (AI) systems, enabling them to perform more efficiently and effectively. One key aspect of AI model optimization is fine-tuning, which involves adjusting the parameters of a pre-trained model to better suit a specific task or domain. Fine-tuning allows AI models to learn from domain-specific data, reducing the need for large amounts of training data and improving their performance on specific tasks. By fine-tuning AI models, businesses can gain a competitive edge in various industries, including customer service, healthcare, and finance.
Fine-tuning leverages the foundational knowledge embedded in pre-trained models, which have been trained on extensive datasets. This process involves retraining the model on a smaller, task-specific dataset, allowing it to adapt to the nuances of the domain. For instance, a pre-trained language model can be fine-tuned with legal documents to enhance its ability to understand and generate legal language, making it invaluable for law firms and legal departments.
The benefits of fine-tuning extend beyond improved performance. By optimizing AI models for specific tasks, businesses can reduce the computational resources required for training, leading to significant cost savings. Additionally, fine-tuning accelerates the deployment of AI solutions, enabling companies to quickly respond to market demands and stay ahead of the competition. In essence, fine-tuning is a powerful tool that transforms generic AI capabilities into specialized, high-performing solutions tailored to meet the unique needs of various industries.
Understanding Fine-Tuning in AI
Fine-tuning is the process of taking a pre-trained AI model—typically trained on vast, generalized datasets—and adapting it to perform specific tasks or meet unique requirements. Utilizing a pre trained llm allows organizations to customize these models for specific tasks or domains. Pre-trained models, such as large language models (LLMs) like GPT or BERT, are designed to handle a broad range of tasks but may lack the precision needed for niche applications. Fine-tuning involves retraining these models on smaller, task-specific datasets to improve their accuracy, relevance, and efficiency for targeted use cases.
For example, a company developing a chatbot for customer support in the healthcare industry might start with a general-purpose LLM. By fine-tuning the model with a training data set that includes domain-specific data—such as medical terminology, patient interaction scripts, and FAQs—the chatbot becomes more adept at understanding and responding to healthcare-related queries. This targeted optimization enhances the model's performance while leveraging the foundational knowledge embedded in the pre-trained model.
Fine-tuning is a critical step in AI deployment because it bridges the gap between generic capabilities and specialized needs. However, its true value lies in its ability to deliver measurable ROI by balancing performance gains with resource efficiency. Monitoring the model's performance during this process is essential to ensure optimal results.
Understanding Large Language Models
Large language models (LLMs) are a type of AI model that has revolutionized the field of natural language processing (NLP). LLMs are trained on vast amounts of text data, enabling them to generate human-like language and perform tasks such as text generation, sentiment analysis, and question answering. However, LLMs often require fine-tuning to perform effectively in specific domains or tasks. Fine-tuning involves training the model on a task-specific dataset, allowing it to learn the nuances of the domain and improve its performance. By fine-tuning LLMs, businesses can unlock their full potential and achieve tangible benefits in areas such as customer interactions and operational efficiency.
The power of LLMs lies in their ability to understand and generate natural language with remarkable accuracy. However, their general training on diverse datasets means they may not excel in specialized applications without further refinement. Fine-tuning addresses this by exposing the model to domain-specific data, such as customer service transcripts or industry-specific jargon, enhancing its ability to generate relevant and accurate responses.
For example, a fine-tuned LLM can be used to develop a sophisticated customer support chatbot that understands and responds to customer queries with high precision. This not only improves customer satisfaction but also reduces the workload on human support agents, leading to increased operational efficiency. Similarly, in the realm of sentiment analysis, a fine-tuned LLM can more accurately gauge customer emotions from social media posts, enabling businesses to tailor their marketing strategies effectively.
In summary, fine-tuning large language models is essential for harnessing their full capabilities. By adapting these models to specific tasks and domains, businesses can achieve significant improvements in performance, leading to better customer interactions, streamlined operations, and ultimately, a stronger competitive position in the market.
The ROI of Fine-Tuning: Why It Matters
ROI is a key metric for evaluating the success of any business investment, including AI initiatives. In the context of fine-tuning, ROI measures the financial and operational benefits of optimizing an AI model against the costs of the fine-tuning process. A well-defined ai strategy is crucial for maximizing these benefits and ensuring that the fine-tuning process aligns with business objectives. These costs include computational resources, data acquisition and preparation, expertise, and time. The benefits, on the other hand, encompass improved model performance, reduced operational costs, enhanced customer satisfaction, and new revenue opportunities.
Key Takeaways:
- ROI is essential for assessing the success of AI investments.
- Fine-tuning costs involve computational resources, data preparation, expertise, and time.
- Benefits include better model performance, lower operational costs, higher customer satisfaction, and new revenue streams.
- A well-defined AI strategy is vital for optimizing these benefits and aligning with business goals.
Fine-tuning delivers ROI in several ways:
- Cost Efficiency: Fine-tuning a pre-trained model is significantly less resource-intensive than training a model from scratch. Training a large-scale AI model can cost hundreds of thousands of dollars in cloud computing resources and require months of development time. Fine-tuning, by contrast, leverages existing models, requiring less data and compute power, which translates to lower costs.
- Improved Performance: Fine-tuned models deliver higher accuracy and relevance for specific tasks, reducing errors and improving outcomes. For instance, a fine-tuned model for a new task like sentiment analysis in social media monitoring can better detect nuanced emotions, leading to more effective marketing strategies and customer engagement.
- Faster Time-to-Market: Fine-tuning accelerates the deployment of AI solutions by building on pre-trained models. This enables businesses to launch products or services faster, capturing market opportunities and generating revenue sooner.
- Scalability: Fine-tuned models can be adapted to multiple use cases within an organization, maximizing the utility of a single investment. A model fine-tuned for one department (e.g., customer service) can often be further refined for related tasks (e.g., sales support) with minimal additional effort.
- Competitive Advantage: By delivering tailored AI solutions, fine-tuning enables businesses to differentiate themselves in the market. A retailer with a fine-tuned recommendation engine, for example, can offer personalized shopping experiences that drive customer loyalty and increase sales.
Key Factors Influencing Fine-Tuning ROI
To maximize the ROI of fine-tuning, organizations must carefully manage several factors that influence both costs and benefits. Training models involves pre-training and fine-tuning large language models (LLMs) to tailor them for specific applications. These include data quality, model selection, computational resources, expertise, and evaluation metrics. A high-quality training dataset is crucial for effective model training, as it ensures the robustness and relevance of the data used in the iterative processes of model training.
Selecting the right base model is also essential, as fine-tuning pre-trained models can significantly enhance performance and efficiency compared to training a new model from scratch.
1. Data Quality and Availability
The success of fine-tuning hinges on the quality and relevance of the data used. High-quality, well-labeled datasets that accurately represent the target task are essential for achieving optimal model performance. Poor-quality data—such as noisy, incomplete, or biased datasets—can lead to suboptimal results, requiring additional iterations and increasing costs. Acquiring labeled data in real-world scenarios can be both challenging and costly, but it is crucial for enhancing performance and accuracy.
To optimize ROI, businesses should invest in robust data collection and preprocessing pipelines. This may involve curating domain-specific datasets, cleaning data to remove inaccuracies, and ensuring diversity to mitigate bias. While data preparation can be time-consuming, it is a critical step that directly impacts the model's effectiveness and, consequently, its ROI. Utilizing the right data, which is high-quality and relevant, is essential for achieving superior AI performance.
2. Model Selection
Choosing the right pre-trained model is crucial for efficient fine-tuning. Not all models are equally suited for every task. For example, a model optimized for natural language processing (NLP) may not perform well for computer vision tasks. Selecting a model that aligns closely with the target use case reduces the amount of fine-tuning required, saving time and resources.
Additionally, businesses should consider the size of the pre-trained model. Larger models often deliver superior performance but require more computational resources for fine-tuning and deployment. Smaller models, while less resource-intensive, may sacrifice some accuracy. Striking the right balance between model size and performance is key to maximizing ROI. Ensuring the model retains valuable pre-trained knowledge while adapting to new data can further enhance its effectiveness.
3. Computational Resources
Fine-tuning requires access to computational resources, such as GPUs or TPUs, which can be costly. Cloud-based platforms like AWS, Google Cloud, and Azure offer scalable solutions, but costs can escalate quickly if not managed carefully. On-premises infrastructure, while potentially cost-effective in the long term, requires significant upfront investment. Training time can also vary significantly based on the methodologies used, such as additive fine-tuning and supervised fine-tuning.
To optimize ROI, organizations should adopt strategies to minimize computational costs. Techniques like transfer learning, which builds on pre-trained weights, and efficient fine-tuning methods, such as LoRA (Low-Rank Adaptation), can reduce resource requirements without compromising performance. Additionally, businesses can leverage spot instances or preemptible VMs on cloud platforms to lower costs.
4. Expertise and Talent
Fine-tuning is a specialized process that requires expertise in machine learning, data science, and domain knowledge. Hiring skilled professionals or training existing staff can be a significant expense. However, the right expertise ensures that fine-tuning is done efficiently, avoiding costly mistakes and rework. Fine-tuning customizes large language models (LLMs) for domain-specific tasks by retraining them on specialized datasets, such as healthcare texts, to improve their grasp of industry-specific terminology.
Organizations can enhance ROI by investing in training programs to upskill their teams or partnering with AI consultancies for specific projects. Open-source tools and frameworks, such as Hugging Face's Transformers or PyTorch, can also streamline the fine-tuning process, reducing reliance on external expertise.
5. Evaluation Metrics
Defining clear evaluation metrics is essential for assessing the success of fine-tuning and its impact on ROI. Metrics should align with business objectives—whether it's improving accuracy, reducing response time, or increasing customer satisfaction. For example, a fine-tuned model for fraud detection should be evaluated based on its ability to minimize false positives and negatives, as these directly affect operational efficiency and financial outcomes. Ensuring the model generates accurate outputs is crucial for maintaining high-quality performance.
Regular monitoring and iteration are also critical. Fine-tuned models may degrade over time as data distributions shift or new use cases emerge. Continuous evaluation ensures that the model remains effective, preserving its ROI.
Human Feedback in Model Optimization
Human feedback plays a crucial role in the optimization of AI models, particularly in the fine-tuning process. Human feedback can be used to guide the model's learning process, providing valuable insights into its performance and areas for improvement. One approach to incorporating human feedback is through reinforcement learning from human feedback (RLHF), which involves training the model to optimize its performance based on human evaluations. RLHF can be used to fine-tune LLMs, enabling them to generate more accurate and contextually relevant responses. By leveraging human feedback, businesses can improve the performance of their AI models, reduce costs, and achieve cost savings in areas such as training costs and data collection. Additionally, human feedback can help ensure that AI models are aligned with human values and goals, reducing the risk of sensitive information being compromised.
Incorporating human feedback into the fine-tuning process allows AI models to learn from real-world interactions and adjust their behavior accordingly. This iterative process helps identify and correct errors, refine model predictions, and enhance overall performance. For instance, in customer service applications, human agents can provide feedback on the accuracy and relevance of the model's responses, guiding further fine-tuning to improve the model's ability to handle complex queries.
Reinforcement learning from human feedback (RLHF) is particularly effective in scenarios where the model's performance is closely tied to human judgment. By continuously evaluating and refining the model based on human input, businesses can ensure that their AI systems remain accurate, reliable, and aligned with user expectations. This not only enhances the quality of the model's outputs but also builds trust with users, as the AI system consistently delivers contextually appropriate and valuable responses.
Moreover, leveraging human feedback can lead to significant cost savings. By fine-tuning models with targeted human input, businesses can reduce the need for extensive and costly data collection efforts. This streamlined approach to model optimization not only lowers training costs but also accelerates the deployment of AI solutions, enabling companies to quickly adapt to changing market conditions and customer needs.
In conclusion, human feedback is a vital component of the fine-tuning process, offering valuable insights that drive continuous improvement in AI model performance. By integrating human evaluations into the learning process, businesses can achieve more accurate, contextually relevant, and cost-effective AI solutions, ultimately enhancing their competitive edge in the market.
Strategies for Maximizing Fine-Tuning ROI
To fully capitalize on the benefits of fine-tuning, organizations can adopt several best practices:
- Start Small and Iterate: Begin with a small-scale fine-tuning project to test assumptions and refine processes. This minimizes upfront costs and allows businesses to learn from early results before scaling up. Additionally, employing few-shot learning can optimize performance when task-specific labeled data is scarce or costly.
- Leverage Open-Source Models: Open-source pre-trained models, such as those available through Hugging Face or Meta AI, provide a cost-effective starting point for fine-tuning. These models are often well-documented and supported by active communities, reducing development time.
- Automate Data Pipelines: Automating data collection, cleaning, and labeling can significantly reduce the time and cost of preparing datasets for fine-tuning. Tools like Labelbox or Snorkel can streamline these processes.
- Use Efficient Fine-Tuning Techniques: Methods like LoRA, prompt tuning, prompt engineering, or adapter layers allow businesses to fine-tune models with minimal computational overhead, preserving resources while achieving high performance. Additionally, supervised fine tuning can be employed to adjust model parameters using a labeled dataset tied to specific tasks, guiding the model to produce accurate predictions.
- Monitor and Maintain Models: Implement robust monitoring systems to track model performance in production. Regular updates and retraining can prevent performance degradation, ensuring sustained ROI.
- Align with Business Goals: Fine-tuning should be driven by clear business objectives, such as increasing revenue, reducing costs, or improving customer satisfaction. Aligning AI initiatives with strategic priorities ensures that resources are allocated effectively.
Conclusion
Fine-tuning is a powerful strategy for optimizing AI models and maximizing ROI. By leveraging pre-trained models, businesses can achieve high performance for specific tasks while minimizing costs and development time. However, realizing the full potential of fine-tuning requires careful attention to data quality, model selection, computational efficiency, expertise, and evaluation metrics. By adopting best practices and aligning fine-tuning efforts with business goals, organizations can unlock significant financial and operational benefits, positioning themselves for success in the competitive AI landscape. As AI continues to transform industries, fine-tuning will remain a cornerstone of delivering value and driving sustainable ROI. Additionally, fine-tuning is crucial for optimizing the deployment of generative AI in various domains.